#51 – Does Big Data Mean Big Evidence?
Episode host: Jason R. Frank.
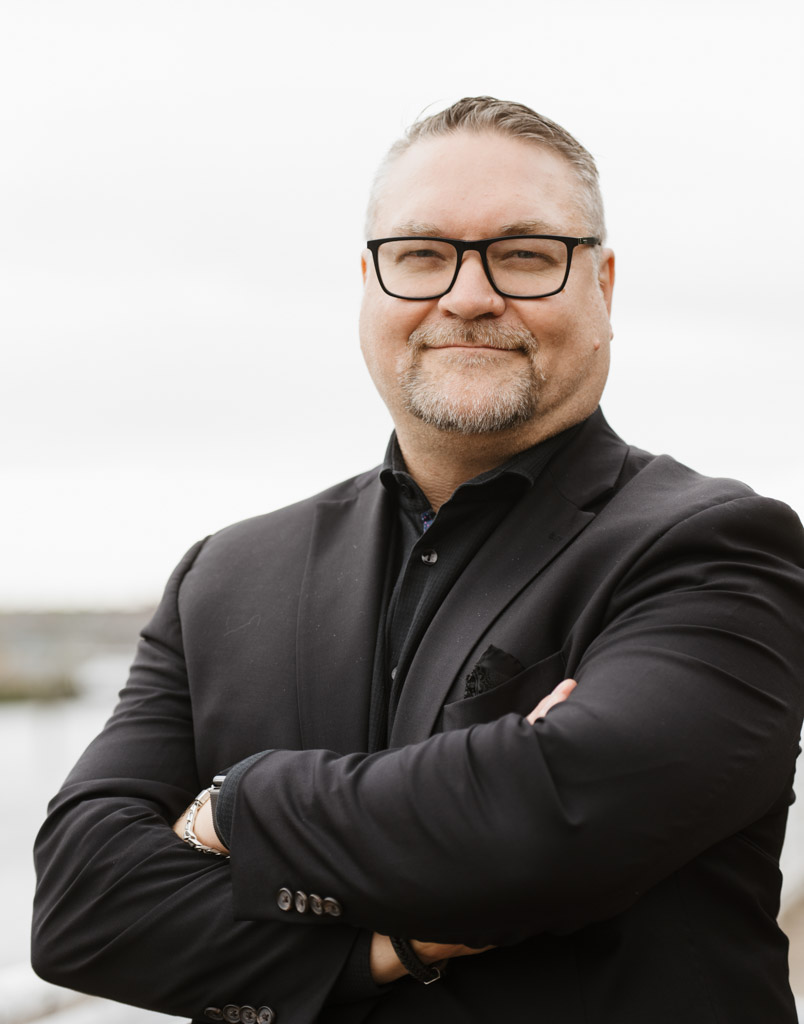
Photo: Erik Cronberg.
Can the calls for evidence-based improvement in HPE be answered by big national datasets?
Episode article
Thelen, A. E., George, B. C., Burkhardt, J. C., Khamees, D., Haas, M. R. C., & Weinstein, D. (2024). “Improving Graduate Medical Education by Aggregating Data Across the Medical Education Continuum”. Academic medicine: journal of the Association of American Medical Colleges, 99(2), 139–145.
Episode notes
Let’s get philosophical for a moment. Do we know what works in HPE/meded? Does our training “work”? Is one institution or curriculum better than another? What does “better” mean anyway?
Background
Our field is growing exponentially in terms of scholarly output, journals, institutions, researchers, innovators, and ideas. Meanwhile, critics have argued that HPE suffers from endless local innovations without advancing an evidence base. The harshest would say HPE is “not scientific”. Counter-critics say to even demand “evidence” smacks of silly positivism, when all education is ultimately embedded in local context. In other words, is there a “right answer” to good practices in HPE?
In the larger view of education globally, these debates continue to rage. Probably, the current consensus is that there are good practices that are always implemented and adapted to a local context.
So what will it take for HPE to advance in a systematic, thoughtful way that is focused on learners, patients, and context?
Purpose
Enter Thelen et al in Academic Medicine in the Feb 2024 issue. The authors describe the product of meetings sponsored by the US National Academies of Sciences, Engineering and Medicine (NASEM) Board on Health Care Services that looked at the prospects of using large national datasets to improve HPE.
More specifically, the authors set out to:
- examine the potential of a national GME data infrastructure to improve Graduate Medical Education (GME)
- review the output of 2 national workshops on this topic, and
- propose a path toward achieving this goal.
Methods
This paper is a narrative consensus report that resulted from workshops held by NASEM in 2017 & 2019. With input from 15 national organizations involved in US GME (PGME), attendees achieved consensus on a desired future using big data to improve HPE. Participants also included leaders from oversight organizations, leaders from teaching institutions, and experts in HPE and big data in healthcare. Examples of existing data collaborations were highlighted and explored.
No other methods were reported.
Results/Findings
The Problem
While the US invests ~$18B per year in residency education alone, there is evidence that graduates continue to have gaps in competence, experience suboptimal training processes, don’t reflect the populations served, and exit with habits that can cause harm. While GME continues to improve, most evidence to inform innovation is based on small innovations without evidence of generalizability.
Quote: “ …lack of access to large-scale data is a key barrier to generating empiric evidence to improve…”
How Large Datasets Can Support Improvement
Large national data could guide innovation in HPE inputs (trainee characteristics and prerequisites), interventions (i.e. curricular designs), and outcomes (shared metrics of outputs of HPE).
Current State
There is currently a lack of comprehensive, aggregated, high-quality, longitudinal HPE data. Each institution in the system collects its own data. Even within institutions there may be siloed data sets by program and phase of career. There are many obstacles to linking these datasets, including: policies, regulations, privacy concerns, database structures and definitions, programming platforms, and tagging of individuals.
Proposed Model Going Forward
The authors propose a new HPE ecosystem in which national data is collected on those involved in HPE, from pre-HP education to practice. Such a data system would need a common data dictionary, standards, and longitudinal links and identifiers. Analysis by individuals, programs, institutions, regions and nationally would enable a new evidence base for improvement. AI and machine learning could inform precision education for individuals to optimize competence. CQI would be enabled. Ultimately, enhanced HPE outcomes would lead to better patient care.
Next Steps.
- Produce an inventory of currently available data.
- Begin data-sharing pilots.
- Begin the work on the technical and governance frameworks needed to share across organizations.
Conclusions
The authors conclude that a national infrastructure effort to link large aggregate HPE datasets will ultimately benefit society.
Comments
- A powerful and inspiring vision of our needed future.
- Very little about the practical barriers and concerns that would need to be overcome.
- Very little from a learner perspective.
0 comments