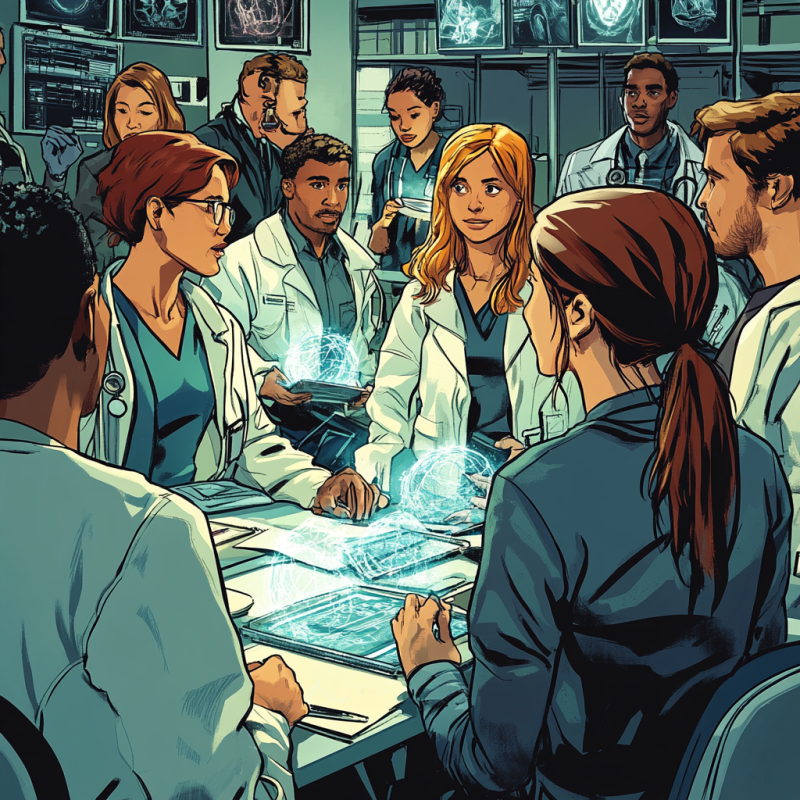
#67 – First we build the AI, then the AI builds us
Episode host: Lara Varpio.
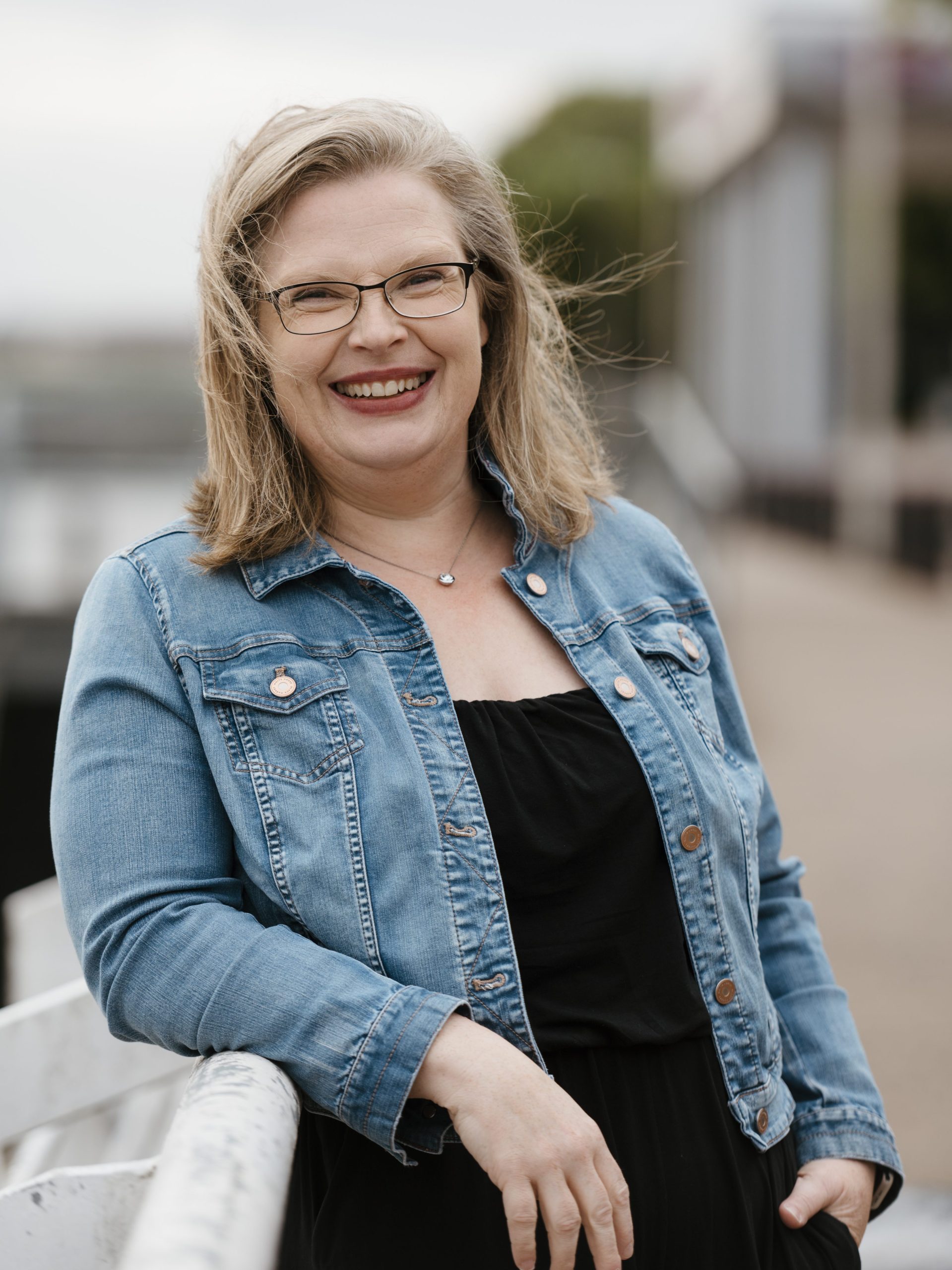
In this episode, Lara leads a conversation about AI and the current body of knowledge about AI that is growing rapidly in Medical Education. Everything you need to know about AI in MedEd is in this paper!
Updated version of the episode out now!
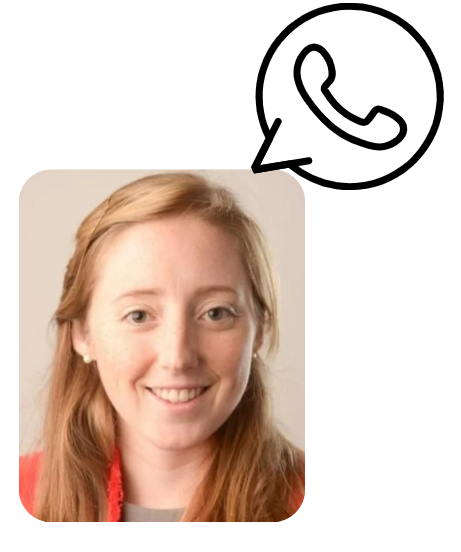
Yes, we made a re-run! What does Hannah Turner, the managing editor for MedEdPortal, have to do with this? Discover for yourself, in this re-run episode.
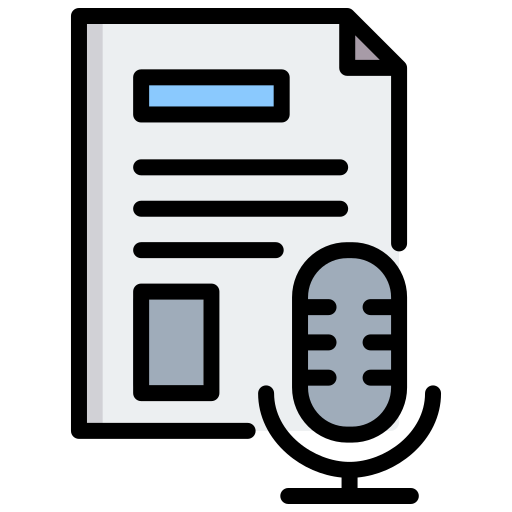
Episode 67 transcript. Enjoy PapersPodcast as a versatile learning resource the way you prefer—read, translate, and explore!
Episode article
Gordon, M., Daniel, M., Ajiboye, A., Uraiby, H., Xu, N. Y., Bartlett, R., Hanson, J., Haas, M., Spadafore, M., Grafton-Clarke, C., Gasiea, R. Y., Michie, C., Corral, J., Kwan, B., Dolmans, D., & Thammasitboon, S. (2024). A scoping review of artificial intelligence in medical education: BEME Guide No. 84. Medical Teacher, 46(4), 446–470.
Episode notes
Here are the notes by Lara Varpio.
Background to why I picked this paper
The paper I picked today is one I found when I was working with a collaborator who is interested in AI and how it is being used in Medical Education. It is the perfect overview paper for all things AI in Med Ed! The paper is entitled A Scoping Review of Artificial Intelligence in Medical Education. It is a BEME review published in 2024
Purpose of this paper
“This review aims to provide insights into the currentstate of AI applications and challenges within the full continuum of medical education.”
(Gordon et al., 2024)
Methods used
The authors conducted a scoping review… with a twist. They conducted a rapid scoping review. From what I could find in the literature about review methodologies, a rapid scoping review is quite similar to a scoping review, but there are a few key differences. One is that the project timeline is short. So if a scoping review is expected to take a year or so to do, then a rapid scoping review aims to be done in 4 months. Scoping reviews have several broad research questions; a rapid scoping review has fewer questions that are clearly specified, narrower. Scooping review has exhaustive and broad searchers; rapid scooping reviews have limits on the scope. Scoping reviews have data extraction approaches that focus on depth and the generation of knowledge; rapid scoping reviews are more tailored in their data extraction that is tailored to meeting specific aims.
This study was conducted within 16 weeks of its inception—so it is a rapid scoping review. They followed Arksey and O’Malley’s methodology. They chose not to use Levac’s recommendation for external stakeholder feedback because they had sufficient expertise on the author team to fulfill that role. They did have 16 authors so maybe…
They searched PubMed/MedLine, Emboss and Med Ed Publish. PubMed and Embase are two good databases that are often used in Med Ed literature reviews. The authors may not have included as many databases as we’d expect, but that’s fine — it is a rapid review. That’s a limit we’d expect to see imposed. They did a hand search of identified articles and added manuscripts that were referenced that were deemed relevant.
They included pretty much every paper published that addressed AI in med ed—excluding only articles that dealt with AI used for diagnostic purposes, clinical or organizational purposes, or that talked about AI only for research purposes They divided the corpus into 2 for data extraction. One group consisted of all the paper that were research reports or innovations. The second group consisted of perspectives and opinion papers. For the research and innovation papers, they developed and then used an extraction tool that collected information about study demographics and characteristics, about how the AI was used, about the kind of AI used, the rationale for the use of the AI, evaluation results or Kirkpatrick outcomes if relevant, and implications for the future. For the perspectives papers, they use the same data extraction tool but also added details about the rational for using AI, its applications framework and recommended topics for curriculum and research, and ethical issues.
Results/Findings
Once the authors did their search and added additional papers found via hand search, they had a corpus to review that consisted of 278 papers. They say a full appendix table of extracted data for all the papers had been uploaded to a repository—unfortunately, it seems that link is broken ☹
You won’t be surprised to hear that, while the first paper about AI was published in 1998, the real surge of papers has come out since 2018. If there were 11 papers published in 2018, that number was up to 57 in 2022 and up to 114 as of August 2023. About 70% of the papers were research or innovation reports, with the other 30% being perspectives and opinion papers. The focus of about 50% of the corpus was UME, 22% was GME, and 3 percent were CPD. With the remainder being some mix of levels. The papers were from 24 different clinical specialities and different basic science departments.
So, there are a TON of different segments to the results. The authors offered many ways of mapping the literature to see what is in there. So instead of me reading all that off to you, we’ll each take a section of the results that we thought was particularly interesting.
Ethics: My interest was piqued by the 14 papers in the corpus that addressed ethics. They expressed caution about the limitations of AI applications in medical education and about the need for educators to really grapple with the ethics of AI when we have yet to really figure out how AI is being used.
The paper lists offered some topics that should be covered in AI ethics education. Some I think are particularly important are algorithmic bias and equity. AI are trained by existing data, and we know those data are non-representative of all populations. This can exacerbate healthcare disparities if we just assume the AI is offering objective facts. It isn’t. It is offering facts generated off biased data sets, so those offerings are biased.
Another ethics concern must be transparency and informed consent. Physicians have a duty to inform their patients about how AI is being used in their treatment and care planning, and about how their data is being collected and used. And their anonymity is not iron clad. With more and more AI resources being developed, it is increasingly possible for people to be identified across platforms.
Conclusions
The paper ends by offering the FACETS framework which the authors suggest should be used to make sure that future manuscripts reporting on AI are comprehensive. This more comprehensive approach, they suggest, can support dissemination, replication, and innovation. The FACETS framework calls for these manuscripts to describe: the form of AI used, the AI use case (ie the end product, innovation, or outcome achieved by the AI), the context, the educational focus, the technology used, and the SAMR (ie the level of technological integration)
“The landscape of AI in medical education, as charted in this review, spans a wide array of stages, specialties, purposes and use cases, primarily reflecting early adaptation phases–only a few describe more in-depth employment for longitudinal or deep change. The proposed FACETS framework is a key outcome, offering a structured approach for future research and practice.”
Re-run of the episode
After this episode first was published (October 1), we got a phone call from Hannah Turner, MPH, Managing Editor, MedEdPORTAL, Association of American Medical Colleges. She wanted to give some additional facts about the platforms and sort things out for us. Big thanks, Hannah, for that. Much appreciated! This and more can be heard in the re-run.
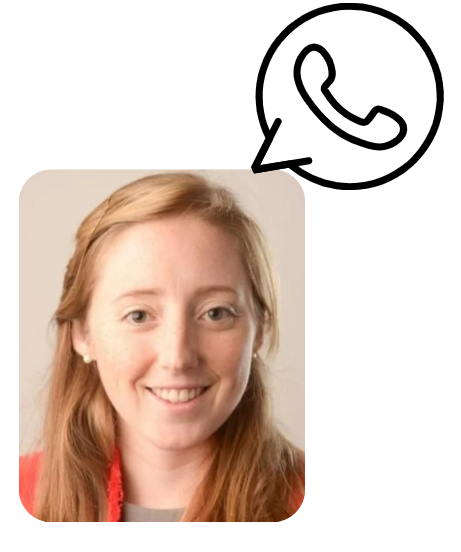
“Hello, my name is Hannah Turner, and I’m the managing editor for MedEdPortal. I’m a new fan of the Papers Podcast after my colleague shared your episode #67 about an AI rapid scoping review where there was some confusion about MedEdPublish versus MedEdPORTAL. In spite of the wonderful real-time fact-checking, I thought it’d be helpful to provide the hosts and listeners with a few additional facts about the platforms.
MedEdPORTAL, The Journal of Teaching and Learning Resources, was established in 2004 and is published by the AAMC. While the article format has evolved over the last 20 years, we’ve always used a traditional peer review model to rigorously assess educational innovations. Our singular publication format includes an article which we call “The Educational Summary Report” and all of the appendices needed to replicate the curricular innovation letter. MedEdPORTAL is led by our editor in Chief Doctor Grace C. Huang, and will soon be led by Doctor Lauren Maggio. Our indexed publications are diamond Open Access, which means they’re completely free to read, submit, and publish.
MedEdPublish was established in 2016 and is published by AMEE. It is a pre-print platform with a post-publication Open Peer Review, after which articles are eligible for indexing. Supported by editorial staff, MedEdPublish has 16 article types that can be published Open Access for an article processing fee. In the end, we find the most similar thing about the two is the name. Happy to answer any other questions at mededportal@aamc.org, and thanks for listening.”
MedEdPORTAL vs. MedEdPublish
MedEdPublish
MedEdPublish is an innovative open-access publishing platform by the Association for Medical Education in Europe (AMEE). It offers rapid publication and open peer review, supporting data deposition and sharing. MedEdPublish focuses on medical education research, including articles, reviews, and case studies, and aims to enhance transparency and reproducibility in research.
Reference: MedEdPublish – How it Works.
MedEdPORTAL
MedEdPORTAL is a MEDLINE-indexed open-access journal of teaching and learning resources in the health professions, published by the Association of American Medical Colleges (AAMC). It focuses on peer-reviewed educational innovations that support medical education, including curricula, simulation cases, and assessment tools.
Reference: MedEdPORTAL website
Comparison of MedEdPORTAL and MedEdPublish
Feature | MedEdPORTAL | MedEdPublish |
Publisher | Association of American Medical Colleges (AAMC) | Association for Medical Education in Europe (AMEE) |
Focus | Peer-reviewed educational innovations | Open-access medical education research |
Content Types | Educational innovation papers; Curricula, simulation cases, assessment tools | Research articles, reviews, case studies, slides, posters, etc |
Access | Free, open-access | Free, open-access |
Peer Review | Yes | Yes, post-publication |
Audience | Medical educators, students, professionals | Medical educators, researchers, practitioners |
Submission Process | Rigorous, structured, free | Rapid, flexible, fee-based (APCs) |
MedEdPublish | Open Access Publishing Platform
Want more? Different aspects of AI in Medical education and Academia can be found in
Papers AI Theme Collection.
Transcript of Episode 67
This transcript is made by autogenerated text tool, and some manual editing by Papers Podcast team. Read more under “Acknowledgement”.
Jason Frank, Lara Varpio, Linda Snell, Jonathan Sherbino.
Start
JASON FRANK: Hi everybody, welcome back to the Papers Podcast. This is Jason, one of the hosts, and I just wanted to let you know that we’re taking a short fall break.
Lara’s probably off writing a paper. Linda’s probably traveling the world. Jon is, who knows what Jons doing. If you know my friend Jon, every week he sort of picks a new project and, you know, one week it’s skeet shooting, the next it’s he’s becoming a sommelier. He’s just kind of, he’s just kind of smart that way. I actually predict he’s doing his nails, something like that.
Manicures are this week’s thing. Either way, we are all off. We hope you’re enjoying the Papers Podcast. Please enjoy the archive. Have a look at our past episodes. Listen to the jokes. Stay for the informative analysis of people’s Papers. As always, we love having you being part of the community. We’ll talk to you again very soon.
[music]
LINDA SNELL: Welcome back to the Papers Podcast. Linda here. And for those of you who listen regularly, thank you for coming. For those of you who don’t listen regularly, you should be, because we…
[gets interrupted]
[talking over each other]
JONATHAN SHERBINO: You’re first here and we’re yelling at you. Get on.
LARA VARPIO: How about a word of welcome. Nice to have you.
JONATHAN SHERBINO: Take your shoes off. Get your feet off the carpet.
LINDA SNELL: What I was going to say was you should be listening regularly because there’s lots of people of the hosts on this podcast who say really important things about Medical Education, like Lara, for instance, who’s going to tell us about her paper.
LARA VARPIO: Hi, everybody. So glad to be here today. And thank you, Linda, for the warm welcome. Jon, how are you doing, hon?
JONATHAN SHERBINO: I’m doing great. I’m fully caffeinated. I have my Father’s Day owl mug rolling. Love that. Yeah.
JASON FRANK: We should describe that for audio listeners.
LARA VARPIO: It’s an owl.
LINDA SNELL: It’s a pink owl.
JONATHAN SHERBINO: It’s an owl. It was painted by a small child at some point. It’s a pink owl. My favorite color. I’m sure my children secretly put lead paint in it because they look at me and say, keep drinking.
LARA VARPIO: Keep drinking from the cup.
JONATHAN SHERBINO: Not long before this is all ours.
LARA VARPIO: And how are you doing, Jason?
JASON FRANK: I’m great.
LARA VARPIO: Sweet. And Linda, you are having a good day in Montreal. The sun’s shining, birds are singing.
LINDA SNELL: Excellent, warm, sunny. I can hardly wait to get outside. So get on with this, will you, Laura?
LARA VARPIO: Let’s do it. So friends, I picked this paper for a reason that’s really simple. I know for our regular listeners, it’s going to come as a shock to hear that perhaps my technological skills are not as high as you might imagine.
LARA VARPIO: And so when I see a paper that summarizes some literature about things that I should know from at the time. The tech side of the house of Medical Education, I’m on that like white on rice. So I have to be honest, though. Like, I’ve embraced chat, GPT. I know how to use it. I can do that.
LARA VARPIO: I am beholden to my navigational apps like Waze and those things because I literally have no sense of direction. So I need that. And as a researcher, friends, if you haven’t played with Scopus AI, you are missing out. You’re. Got to get out on Intascopus. It has an AI function. It’ll change your life.
LARA VARPIO: So when I saw this manuscript, I’m like, yes, I need this. I appreciate AI, but like there’s a bunch of Papers in the field now and I need somebody to help make sense of it. Ergo, I picked this paper. The paper is entitled A Scoping Review of Artificial Intelligence in Medical Education. It’s a BME review published just this year, 2024.
LARA VARPIO: Now, before we get into the meat of the paper, I’m curious about how all of you have adopted. AI into your Medical Education practices. And I’m okay with the fact that maybe you haven’t, but have you seen your learners and colleagues using it in interesting ways? What has surprised you about AI to date?
LARA VARPIO: Jon, I’m going to make you go last because I’m pretty sure it’s possible, like if it was possible to literally mainline the technology into your veins, you’d be like, move over seven out of nine, I’m plugging into the Borg. So I’m going to make you go last. Let’s go Jason, Linda, JJon
JASON FRANK: I’m glad you asked me first because I have a quick question for you. Why is this a beanie review? It’s a scoping review, which is kind of like, you know, here’s a picture of the landscape. But it doesn’t strike to me like it should be a beanie review, which is best evidence available. What’s your take on that?
LARA VARPIO: So my take on that is and I’ll be really interested with the rest of y’all thing, because my understanding is that be me is an organization that’s A.
LARA VARPIO: Don’t ask me why I feel it’s affiliated with Amy or the specific way in which it’s affiliated because I don’t know the specifics there. But I believe it’s affiliated with Amy. And the idea behind this Be Me group organization is that they help to make sure that knowledge syntheses that are done in Medical Education are done in a robust kind of way.
LARA VARPIO: So if you do a scoping review or a systematic review, you can work with or apply to Be Me to have somebody look over your materials, over your context, over your… Your processes to make sure that what you generate actually is best evidence. That’s my understanding.
JASON FRANK: It’s like a stamp from a group as opposed to this is best evidence. It’s just a name.
LARA VARPIO: Maybe. That’s your take on it? I don’t know. I don’t know.
JASON FRANK: Okay. So back to your question, AI, I am still a dabbler. My kids are in this house in their 20s. AI automates a lot of things. I’ve started to automate some things, not as much as Jon. And I got to tell you, though, when I’ve used AI as in part of searches, I get a lot of hallucinations.
JASON FRANK: So that kind of turns me off, but I’m working on it.
LINDA SNELL: I use the navigation aids and I use ChatGPT for myself. But I’d like to talk about how my students use AI, usually ChatGPT and usually when they’re writing a paper. And often, but not always, when they’re writing in a language that’s not their first language.
LINDA SNELL: And sometimes what comes out hasn’t been checked. And just the same as Jason gets hallucinations. Students often get hallucinations and they don’t check or don’t know to check what’s coming out. And so sometimes you get really weird things in their assignments.
JONATHAN SHERBINO: All right. I got lots of things to say. First, I love, love, love the Star Trek reference. Thank you very much. Buddy!
LARA VARPIO: Totes!
JONATHAN SHERBINO: There we go.
LARA VARPIO: Oh yeah, live long and prosper.
JONATHAN SHERBINO: So for those of you who think that there’s hallucinations, you’re using old generation. Those hallucinations are going way fast. And you’re… There’s a lag in your own human brain with what’s actually happening. In terms of search, I’m going to direct you to Illicit. Have you tried that, Lara?
LARA VARPIO: No, I don’t know Illicit.
JONATHAN SHERBINO: I don’t have any stakes in the company, so this is not A…
LARA VARPIO: It does sound polite, though. Can we talk about it on the podcast?
JONATHAN SHERBINO: It makes it even more exciting.
LARA VARPIO: Okay.
JONATHAN SHERBINO: But I would love to…
JASON FRANK: Is it Explicit or Illicit?
LARA VARPIO: I heard Explicit, so…
JONATHAN SHERBINO: It does great work summarizing and searching for you in terms of technical Papers. My new writing hack… Is I will dictate into a document and I get a flow of consciousness and I get past writer’s block and I do all that and then I dump it into an LLM and it cleans it for me and it comes back in workable text prose.
JONATHAN SHERBINO: If you’ve ever listened to a transcription of a conversation, you’re like, this is just junk when you read it. But if you can just talk your way to the first draft and then have Gen AI clean it and then you actually have a workable first draft, that’s really exciting in terms of just workflow.
JONATHAN SHERBINO: But the last part is actually I’m founding a startup that’s using NAI.
LARA VARPIO: Are you really?
LARA VARPIO: Count me in. I don’t know what we’re doing, but I’ll do it.
JASON FRANK: Are you surprised?
JONATHAN SHERBINO: I have an NDA you’ll need to sign. Actually, I have an NDA for all the listeners they have to sign before I can tell you more. But I think this is an interesting way to advance teaching with a transformation of the type of teaching we’re doing rather than just an augmentation.
JONATHAN SHERBINO: We’re going to talk about transformation and augmentation. Later in this podcast, because I think there’s a really great framework for adjudicating new educational technologies. So yes, it’s here. Welcome Skynet. Take me up. I’m ready. I’m ready. I’m ready for the cable to the back of my head.
LARA VARPIO: Beam me up, Scotty. All right. So this paper, as I said, is a beamy scoping review. And I just want to say that the author list is long. Many people you know are on the author list. First author is Morris Gordon.
LARA VARPIO: And the The goal of the research, and I’ll just read it for you, this review aimed to map the literature regarding AI applications in Medical Education, core areas of findings, potential candidates for formal systematic review, and gaps in the literature for future research. Fine.
LARA VARPIO: So let’s dive into the methods. The authors conducted a scoping review with a twist. In the abstract, they call it a rapid scoping review. In the first line of the method section, they say the scoping review was conducted in rapid timeframe.
LARA VARPIO: So this led me to a lovely afternoon of reading about methodologies of rapid reviews, because I don’t know much about rapid reviews and rapid scoping reviews more precisely. Let me share with you just a little snippet of what I learned. From what I can find, a rapid scoping review is really quite similar to a scoping review, but there are a few key differences.
LARA VARPIO: One is that the project timeline is short. So if a scoping review is expected to take a year or so, maybe more to do, then a rapid scoping review is closer to four months. If scoping reviews have several broad research questions, a rapid scoping review is going to limit that scope.
LARA VARPIO: And if scoping reviews have data extraction approaches that focus on depth and generation of new knowledge, then a rapid scoping review, those questions are more tailored in the data extraction and trying to meet a very specific aim, probably a narrow one. So this study was conducted within 16 weeks, like, wow, of its inception, 16 weeks from the day we started to the day we’re done. So that’s pretty rapid, for sure.
LARA VARPIO: They followed Arksey and O’s methodology, fine. They chose not to do Levesque’s recommendation for external stakeholders because they felt they had sufficient expertise in the author team. Hear a comment previously, look at the author list, you’re going to know these people. I’m okay with that. They searched PubMed, Medline, Embase, and MedEd Publish.
LARA VARPIO: Now, for the record, I did think that was a bit odd because PubMed and Embase, that’s fine. Yeah, good databases, but maybe not as many as we’d expect, but it’s a rapid review, fine. But MedEd Publish, I’d be interested in what you thought about that because MedEd Publish is a post-production review platform, right? So, MedEd Publish? Which one’s MedEd Publish?
JASON FRANK: This is the AIME alternative open access platform.
JASON FRANK: No. Right? It’s like, what’s the one in AAMC runs? It’s a repository of educational objects. Come on, guys, help me here.
LINDA SNELL: MedEd Portal?
JASON FRANK: MedEd Portal. I think of it like the MedEd Portal.
LARA VARPIO: But MedEd Portal is different than MedEd Publish, rig ht? Because MedEd Publish is the post-production review, right?
LARA VARPIO: No, I think it’s-Jon, I’m looking at you.
JASON FRANK: It’s an alternative platform.
LINDA SNELL: So are these peer reviewed? What’s on there? Yes.
JASON FRANK: Yes.
LARA VARPIO: Okay. We’ll discuss this more.
JASON FRANK: Readers can help us out, but go ahead and tell me everybody that I’m right.
LARA VARPIO: No, I’m not going to go with that. What I’m going to do is I’m watching my friend Jon go online and sort this out right now because he’s not going to let us have this hanging.
JASON FRANK: Jon gets all the cred.
LARA VARPIO: What the hell? Because he’s good. No, he is. But I mean, from a technology side. Look at us.
JASON FRANK: It’s a thought effect issue.
LARA VARPIO: Well, I’m just saying he’s a public thing. He’s recording and he’s doing a search. I’m not sure the other three of us can do two things at the same time. Moving on.
JASON FRANK: ….
JONATHAN SHERBINO: All right, so-Here it is. MedEd Portal, you put an innovation online and you actually share the innovation. You share the curriculum, you share the tool, you share the cases, and that’s funded by AAMC.
LARA VARPIO: And that’s that. Which one is that one?
JONATHAN SHERBINO: MedEd Portal. Portal. MedEd Publish.
LARA VARPIO: Yes.
JONATHAN SHERBINO: Cheers.
LARA VARPIO: Amy.
JONATHAN SHERBINO: Rapid, funded by Amy. It’s a rapid, transparent publishing site that does have peer review. It would look much more.
LARA VARPIO: But peer review is after publication, isn’t it?
JASON FRANK: No.
JONATHAN SHERBINO: So it’s exactly like Curious, which is an open access resource. So sadly, I’m going to have to agree with you on this one.
LARA VARPIO: All right. Come on. Anyway, I feel you may have had a partial point. But anyway, but my point here is that I just, I found myself having a whole moment of, okay, database, database, and what? But anyway, that’s fine. Let’s talk about it. I’d be open to your conversations and comments about that selection.
JONATHAN SHERBINO: Now, peer review of articles takes place after publication. The articles published.
LARA VARPIO: Shut the front door! Varpio for the win! [large explosion sound effect]
JONATHAN SHERBINO: I wasn’t going to let Jason win. Don’t worry. I got you.
LARA VARPIO: I love you. I love you.
JASON FRANK: So wrong.
JONATHAN SHERBINO: But you have to get an expert reviewer and you invite them to review it. And then they put their review online beside your paper.
LARA VARPIO: Okay. So I post my paper. They post it without review. I find a friend and say, could you please go say nice things about my paper on the website? And then they do.
JASON FRANK: Yeah.
HANNAH TURNER: Hello, my name is Hannah Turner and I’m the managing editor for MedEd Portal. I’m a new fan of the Papers Podcast after my colleague shared your episode number 67. About an AI rapid scoping review where there was some confusion about MedEd Publish versus MedEd Portal.
HANNAH TURNER: In spite of the wonderful real-time fact-checking, I thought it’d be helpful to provide the hosts and listeners with a few additional facts about the platforms. MedEd Portal, the Journal Of Teaching And Learning Resources, was established in 2004 and is published by the AAMC.
HANNAH TURNER: While the article format has evolved over the last 20 years, We’ve always used a traditional peer review model to rigorously assess educational innovations. Our singular publication format includes an article, which we call the Educational Summary Report, and all of the appendices needed to replicate the curricular innovation.
HANNAH TURNER: MedEd Portal is led by our Editor-in-Chief, Dr. Grace Wong, and will soon be led by Dr. Lauren Maggio. Our indexed publications are diamond open access, which means they’re completely free to read, submit, and publish.
HANNAH TURNER: MedEd Publish was established in 2016 and is published by AMEE. It is a pre-print platform with a post-publication open peer review, after which articles are eligible for indexing.
HANNAH TURNER: Supported by editorial staff, MetaPublish has 16 article types that can be published open access for an article processing fee.
HANNAH TURNER: In the end, we find the most similar thing about the two is the name. Happy to answer. Any other questions at mededportal at amc.org. And thanks for listening.
LARA VARPIO: Back to the authors and this literature review.
LARA VARPIO: They did a hand search of identified articles and added manuscripts that were cited that they deemed relevant. They included pretty much everything published that addressed AI and MedEd that I would have of my collection of articles. All of them were in there. So, yeah. Excluding only articles that dealt with AI for diagnostic purposes, clinical or organizational purposes, or that talked about AI only for like a research.
LARA VARPIO: They divided the corpus into two into two for data extraction. One group. Consisted of all the Papers that were research reports or innovations. The second consisted of perspectives and opinion Papers. For the research and innovation Papers, they developed and then used an extraction tool that collected information about study demographics, how the AI was used, about the kind of AI used, the rationale for it, etc.
LARA VARPIO: For the perspective Papers, they use the same data extraction tool, but also added details about the rationality, the rationale for using AI, its application of the framework. And recommended topics for curriculum and research in this edge. So while we’ve had a robust discussion about all kinds of things that are not relevant, I would be very interested in your thoughts on the methods. Let’s go, Linda, Jason, Jon.
LINDA SNELL: So I love the fact that they had sort of a double-pronged thing. If you look at most lit reviews, they exclude opinion Papers and perspectives and things like that. And I think for this particular research question, it was really important to have those. In there for this type of a scoping review. So good on you guys for having that two-pronged part on innovation and part on perspectives.
JASON FRANK: You know what? If you accept that it’s a rapid, rapid review, it’s right on the money. And they also get extra marks from me for the nice, colorful diagrams. Even Jon would like these infographics, and he’s pretty critical.
JONATHAN SHERBINO: This is an exemplary rapid scoping review. They follow the Prisma extension. They have a large author group, which you might wonder, why do they have 16 authors on this paper? It’s because they have a lot of work to do. They have to do it quickly, but they also need to synthesize the literature. That’s they have wrapped their arms around a big corpus of studies and, editorials and commentaries.
JONATHAN SHERBINO: Their search ends August of 23. They submit December 23. They’re accepted January 24, and they’re published in an in a volume, April 24.
JONATHAN SHERBINO: No wonder they have 16 authors. I have no concerns about any methodologies. I’ll pay attention to their results because I don’t think there’s a critical or even a minor flaw here.
LARA VARPIO: Knowing that they had 16 authors and listening to that timeline, do you know what boggles my mind? I have a hard time getting all like shorter author lines, like fewer people. I have a hard time getting all those ducks in a row for the paper. They went from finishing the review to writing the paper in a really short time.
LARA VARPIO: So to the lead author, congratulations on the leadership skills because that’s legit. Okay, moving on to results. How many Papers did they end up with? Brace it. Brace for it. Hold on tight for 278. Shoot me now. That’s a lot. And now we remember why they had 16 authors. But, you know, respect. They did this in 16 weeks.
LARA VARPIO: They say a full appendix of extracted data for the Papers had been uploaded in a repository. While the first paper about AI published starting in 1998, the real surge of Papers came around 2018. If there were 11 Papers published in 2018, that number was up to 57 in 2022. And it was at 114 as of August 23.
LARA VARPIO: So, yeah, it’s a hot topic. About 70% of Papers were research and innovation. The other 30, perspectives and opinions. The focus of about 50% of the corpus was undergraduate Medical Education, leaving 22% for GME and only three for CPD. And we’ve already thought about how this is good. Go ahead, Linda, you want to say something?
LINDA SNELL: I was really, in a way, disappointed that only 3% was CPD. I wasn’t surprised, because I think that that’s where we need to focus our attention when it comes to, you know… Being efficient in education for people in practice.
LARA VARPIO: Yeah.
JASON FRANK: I wonder if that’s just proportional to the whole med ed literature. There’s fewer Papers about CPD.
LARA VARPIO: Fact. Okay. But the Papers were from 24 different clinical specialties, basic science departments. So, you know, long story short of the demographics, everybody’s talking about it. Everybody is in on this conversation. So when you get to the results and you see like the technical term is a bucket ton of different segments for the results.
LARA VARPIO: That’s why. Because there’s 278 articles. They found many different ways of mapping the literature. So instead of me reading all of that to you, what we’ve done amongst the four of us is we did some homework and we all picked a section. The part that I thought was really cool was about the ethics. So my interest was piqued by those 14 Papers in the corpus that addressed ethics.
LARA VARPIO: They talk about a caution of the limitations of AI applications in Medical Education and about the need for educators to really grapple with the ethics of AI when, let’s be honest, most of us are still trying to figure out how to use it, what it is, what we do. It’s a real moving target that we’re actively in the midst of developing.
LARA VARPIO: So ways of using AI are… Constantly being developed. And so we need to be constantly reflecting on the ethical questions that those evolutions and developments bring with them. The paper list offers some topics that should be covered about AI ethics. Some of the ones I thought were particularly important are algorithmic bias and ethics or equity, right?
LARA VARPIO: So what data is in there makes a big difference on what comes out, what’s generated by the AI. And we know that the knowledge going into the AI, there’s implicit bias, there’s structural inequity embedded in that. So we really do need to be worrying about that.
LARA VARPIO: The second one I just want to highlight is about transparency and informed consent. Physicians have a duty to inform their patients about how AI is being used in their treatment and care planning, and about how the data is being collected and used. Their anonymity is not ironclad, right? With more and more AI resources being developed, it’s getting increasingly possible for people to be identified across platforms.
LARA VARPIO: So we really got to be thinking and asking hard questions about what data am I generating? How am I sharing it? And I also have to ask questions about the AI that’s the generation that the product that’s generated by AI, is it really an equitable, just product? Or do I have to ask some questions here?
LARA VARPIO: So with that, that’s my view on the ethics piece. I’m interested in what you’re thinking. So let’s go, Jon, Linda, Jason.
JONATHAN SHERBINO: First off, in terms of a scoping review, the results are typical of a scoping review. At the start of the review, there’s very little. And right at the end of the search, there’s a lot because you choose a topic that is timely and of interest and it builds over time.
JONATHAN SHERBINO: Nobody’s saying, hey, I wonder what the lecture of the professor in… An operating theater looks like because that’s what we did 200 years ago. So that kind of feels the same. We also see this similar typical demographics around geography. There’s over-representation of the global north versus the south.
JONATHAN SHERBINO: That probably speaks to constraints and resources. And then the last part is we don’t see continuing professional development really included in this conversation. Our education scholarship is heavily focused on UGME and then subsequently on PGME. And we forget where I think AI might have the biggest…
JONATHAN SHERBINO: Opportunity. It’s the cultivation, it’s the just-in-time, it’s a very tailored distribution to a busy clinician in practice. That’s an interesting gap for me. Now, I want to talk about assessment. And as I kind of alluded to before, I want to talk about this SAMR EdTech model, which has been used in a number of, I’m not sure if we’ve talked about it on the Papers Podcast before, but it’s a fairly well-known model.
JONATHAN SHERBINO: And it stands for substitution. So how can a technology replace a traditional method? Augmentation, how can a technology bring a new functionality to an existing process, modification, how does it redesign or improve a task, and redefinition, does it bring something completely new into the conversation.
JONATHAN SHERBINO: And I think when you use this type of framework, it helps you say what’s happening with technology. It’s not all apples, it’s more fruit salad. It’s tweaks or it’s complete change or it’s replacement. There are… Our computer overlords have made us redundant as assessors, which might be a cheer from some of the people on the podcast.
JONATHAN SHERBINO: Let me give you some examples, but there’s a richness of it. It seems that the literature is very heavily at the substitution. We take out menial tasks using AI for assessment or augmentation. We take a task, but we make it a little bit more streamlined. And I’ll give you some examples. So at substitution, there’s a whole bunch of Papers around multiple choice question generation.
JONATHAN SHERBINO: At augmentation, there is the video analysis of psychomotor skills. So can AI see how you’re doing and produce a parallel score for a human assessor? Or can human assessors provide narrative feedback and then the AI actually scrape that data, analyze it, and provide summation of all of the narrative scores for an individual? And so… That’s an example of one of the studies that we perform.
JONATHAN SHERBINO: So can you take 20,000 words of narrative assessment over the course of residency training and say, here’s the trajectory, here’s the arc? At the modification level, can you take a task and redesign it in a significant way? So one example is the development of a virtual OSCE, which also has online grading. And so we take something and we transform it in a way.
JONATHAN SHERBINO: And then at the redesign or the redefinition or the redesign level is, can we predict medical students’success on high-stakes exams when we dump all of our assessment data into it? And produce true learning curves or true future performance models. That was the holy grail of programmatic assessment 10 years ago. And maybe with AI, we might be able to get through the noise to actually see some data.
JONATHAN SHERBINO: So I think, and I’m a big positivist for AI, I think there’s lots of opportunity here to take our role of assessment and substitute some of the meaning.
JONATHAN SHERBINO: Meaningless work that we do augment and so we get better outputs modify meaning we change our current models but turn it into something that gives us new ways of thinking or completely redefine how we’re going to assess so i’m i’m pretty bullish on on where we’re going with AI and assessment ps love the graphics in this study i don’t say that often so ps love the graphics there are a lot of There are a lot of clip art, but they did it in a good way.
LINDA SNELL: Yeah, they got lots of primary colors, Jon. That’s good for you to play with. Get your crayons out. I picked the section on admissions and selection and the use of AI there. And I first started thinking, what are some of the challenges in admissions and selection? And there’s a couple that come to mind. One is bias.
LINDA SNELL: The second is, oh, I’ve got a huge number of individuals to look at, the large numbers. The third is. Am I making the right decisions? In other words, the prediction. And the fourth, which is a very practical one, is everybody’s asking me the same questions when they come for the interviews, whatever they’re coming for.
LINDA SNELL: Can I deal with that to make things more efficient? So when you look at some of the AI Papers, first, the AI has been used to host a question and answer session. I presume this was for… Candidates who are all asking the same questions. And so that’s obviously very efficient. Second, AI used to detect a gender bias in letters of reference.
LINDA SNELL: And in fact, there was a gender bias in letters of reference. Third, AI used to rank medical student evaluations and comparing that with faculty evaluations. And in this case, AI failed. It didn’t pick up the nuances and the subtleties. Fourth, as a predictive model, who was ranked and matched. And the success in that was very high. AI could be very helpful there.
LINDA SNELL: But most of the studies had to do with screening applications when you have large numbers of applicants. And AI was very successful for that. It also identified applicants who might otherwise…
LINDA SNELL: Have fallen through the cracks, who might otherwise have not been chosen because of a bias. So bottom line is, I think if you think about the challenges you have in a particular area and how AI can help, in general, AI can help with admissions and selections.
JASON FRANK: Cool. I’m also very enthusiastic about this paper. When I first looked at it, I thought, oh, this is going to be a giant list, a long smorgasbord of every paper. But actually, it’s really good. It’s really accessible. It’s going to be highly cited.
JASON FRANK: Let me highlight one area which I think has potential. So all of you, I know Jon’s seen that. All of you have watched the reboot of Star Trek that came out a few years ago. And there’s a scene, JJons doing like Peace and Prosper.
JASON FRANK: There’s a scene where a young Spock in this reboot is in this pit. Yes. And there’s an AI interacting with Spock. Teaching, correcting, giving more challenging questions, kind of like it’s responsive to his right answers and so on. So isn’t that cool?
JASON FRANK: That’s a section in this paper that you can teach clinical reasoning using AI that is tailored, can generate a whole bunch of a library of clinical cases with key features and help a given learner recognize key features, get more accurate, be even smarter. I think that’s got a lot of potential and it’s probably the future.
LARA VARPIO: So just for the record, listeners. Star Trek isn’t in the paper is just like a common trend, apparently, throughout our conversation today. So don’t be disappointed when you don’t have a figure of Spock in the hole with the teacher outside, because there’s lots of other figures in the paper that are excellent.
LARA VARPIO: So I’m just going to end with a few words about the discussion. Two things specifically. One, the paper ends by offering what they call the facets framework.
LARA VARPIO: And the facets framework is all about trying to make sure that all the Papers that continue in the future cover, they’re comprehensive, they cover all the aspects of the AI that we want to know about so that we can do cross comparisons as this literature grows and develop greater insights, making synthesis probably easier.
LARA VARPIO: So you can kind of imagine how the facets framework came into mind. It’s not because what their job was easy, right? Papers were missing important pieces. I encourage the listeners to go and look at that framework.
LARA VARPIO: And if you’re going to write about an AI innovation, I think it’s probably a good idea to look at the facets framework just to say, did I address and mention and report all of these things? Go ahead. Sherbino has got another interruption.
JONATHAN SHERBINO: I just wanted to endorse what you’re saying. We’re at the period where everyone has something new and shiny, but at some point we need to mature this literature. And so Dave Cook would remind us that comparative effectiveness research is really important.
JONATHAN SHERBINO: So you have to say, okay, And using that framework, the facets framework allows you to tag or to categorize what you’re actually doing so that the next iteration is not just a new thing and that we don’t just keep seeing shiny baubles everywhere.
JONATHAN SHERBINO: We try to advance MCQ generation forward in a way. We try to advance video OSCEs forward in a way. And you can’t compare it just on the superficial features. You need this framework that you’re describing.
LARA VARPIO: Linda?
LINDA SNELL: I agree. When I first looked at the framework, I poo-pooed it. I said, ah, this is very generic. It’s not going to help. And then I realized it has to be generic because it’s addressing the broad field of AI. And so, yeah, I think it’ll be helpful.
JASON FRANK: But couldn’t it be used, honestly, for any innovation? Like you just adapt the wording a little bit. So it’s not about AI. It seems really useful actually that way.
LARA VARPIO: We have had a great conversation. I’m excited to get to our voting because we’re all going to agree it’s a five across the board on both items. So let’s start with methods and we will go Jason, Linda, Jon.
JASON FRANK: Methods are good. Four.
LARA VARPIO: Linda?
LINDA SNELL: They’re good, but more than good. Five.
LARA VARPIO: Jon?
JONATHAN SHERBINO: Can I just say that for the first time in forever, you forced us to use an ordinal scale. And I’m so happy. And there’s going to be no…
LARA VARPIO: I’m going to give it a 4.5, just to drive you.
JONATHAN SHERBINO: It’s a 5.
LARA VARPIO: I’m going to give it a 4 and a Spock. Boom shakalaka.
JONATHAN SHERBINO: Oh, man. Why did I just push your buttons? It’s a 5. This is great methodology.
LARA VARPIO: And now to results. One out of one to five. Let’s go inverse. Jon, Linda, Jason.
JONATHAN SHERBINO: Mine’s a 5 with an asterisk. And the asterisk is.
LARA VARPIO: Oh, and you’re giving me a hard time for not doing ordinal whatever.
JONATHAN SHERBINO: This is going to stale date very quickly. Yeah. So right now, this is state of the art. This changes everything. But read this paper in two years. You’re like, yeah, I know all this. And so read it now and tell all your friends now.
JONATHAN SHERBINO: But don’t put it in your reading list to get to it in a future date because then you’re like, oh, this is just a treat. Or this is what it looked like 18 months ago. This is Moore’s Law happening right now.
LINDA SNELL: Yeah, I agree it’s a five now, but I wouldn’t give it two years, Jon. I’d give it six months before it’s stale dated.
LARA VARPIO: Yeah, lots of Papers coming out. Jason?
JASON FRANK: I think it’s a five because people will refer to this for the next year or so, and you can reference it to launch any AI paper, and facets is useful.
LARA VARPIO: And I too am going to give it a five for all the reasons mentioned. And so with that, friends, and just because Jon was so excited I gave it a five, I’m going to give it a five and an asterisk. And I’m not going to tell you what the asterisk is for, and that’s going to drive you nuts.
LARA VARPIO: So friends, thank you for listening to yet another recording of the Papers Podcast. We’re so glad you’re here. And because we want to hear from you, we are going to ask you to get in touch with us. And because it’s a skill that is like a Vin Diesel level skill, Jon’s going to tell you how to get in touch.
JONATHAN SHERBINO: You couldn’t remember, could you?
LARA VARPIO: No, I couldn’t remember.
JONATHAN SHERBINO: You can reach us on our website, paperspodcast.com. Or if you’re Vin Diesel and you still use the email. And you’re fast and furious with your typing on email. You can reach us at thepaperspodcast at gmail.com.
JONATHAN SHERBINO: I did want to give a shout out to one of our listeners. I think a new listener to the Papers Podcast, Suraj Mithawati. Suraj is a hematologist here at McMaster. And he wrote us a really nice note. We covered one of his Papers. I can’t remember which episode it was. It was a paper on practice variability. And his comment is, it’s always been one of my nerd goals to have a paper featured on the podcast.
JONATHAN SHERBINO: Love the discussion and learned a lot from it. I’m really grateful for that kind of comment when we can have a value add to the authors, let alone the listener. So thanks, Saraj, for the note. Thanks for also taking my calls in the middle of the night when I’m saying, the CDC looks all crazy. What do you think it means? Thanks for listening.
LARA VARPIO: Talk to you later.
JASON FRANK: Take care, everybody.
LINDA SNELL: Bye-bye.
JASON FRANK: You’ve been listening to The Papers Podcast. We hope we made you just slightly smarter. The podcast is a production of the Unit For Teaching And Learning at the Karolinska Institute. The executive producer today was my friend, Teresa Sörö.
JASON FRANK: The technical producer today was Samuel Lundberg. You can learn more about The Papers Podcast and contact us at www.thepaperspodcast.com. Thank you for listening, everybody. Thank you for all you do.
LARA VARPIO: Take care.
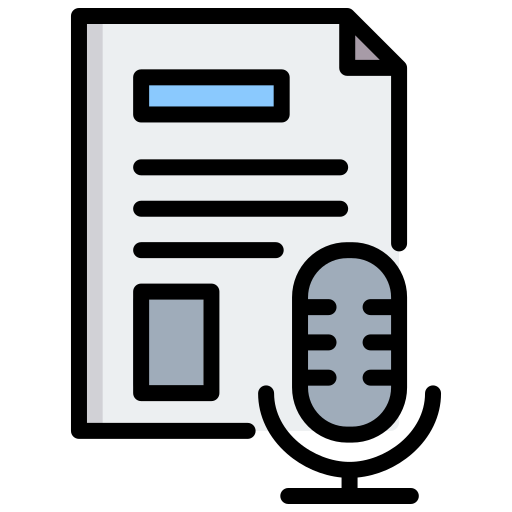
Acknowledgment
This transcript was generated using machine transcription technology, followed by manual editing for accuracy and clarity. While we strive for precision, there may be minor discrepancies between the spoken content and the text. We appreciate your understanding and encourage you to refer to the original podcast for the most accurate context.
Icon designed by Freepik (www.freepik.com)
0 comments