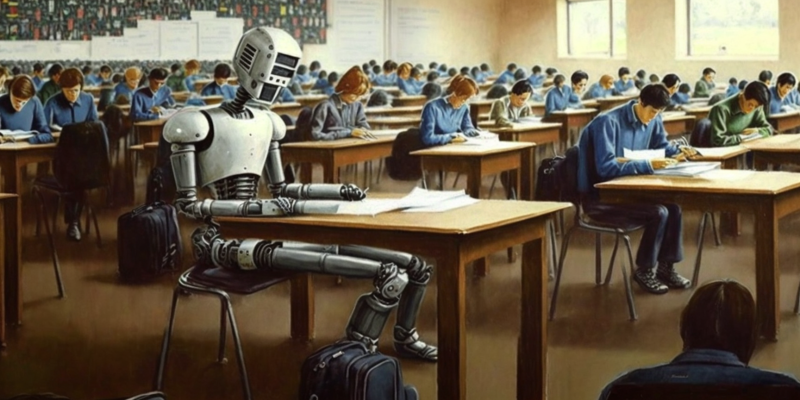
#10 Ex machina: The disruption of HPE with AI
In this episode we examine the feasibility of a hugely popular chatbot to answer a national medical licensing exam and discuss the implications of this disruptive innovation. Chatbots use natural language processing (NLP) to converse and answer questions posed by a human user. Large language models (think billions of language parameters/nodes connected via networks to produce non-linear correlations between nodes) have accelerated the usability of chatbots. Original composition, answering complex questions etc. are some of the features.
Host: Jonathan Sherbino.
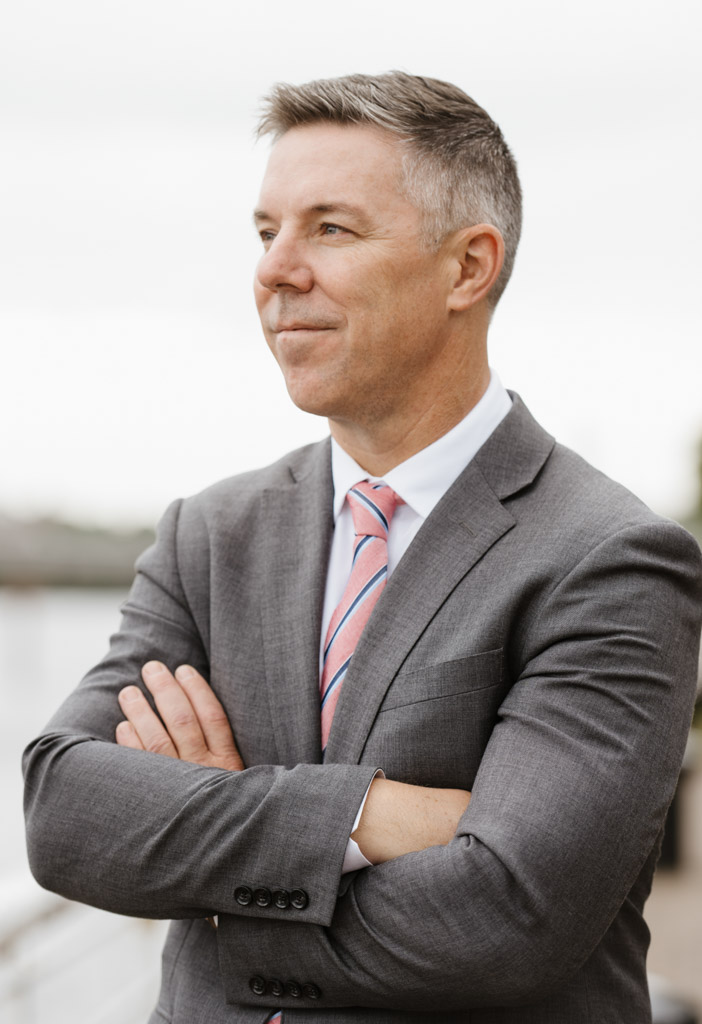
Enjoy listening to us at your preferred podcast player!
Apple Google Spotify Spreaker
Episode article
Gilson, A., Safranek, C. W., Huang, T., Socrates, V., Chi, L., Taylor, R. A., & Chartash, D. (2023). “How Does ChatGPT Perform on the United States Medical Licensing Examination? The Implications of Large Language Models for Medical Education and Knowledge Assessment“. JMIR Medical Education, 9(1), e45312.
Background
HPE is being disrupted. Don’t believe me. Ask a student. Ask your kids. Ask a teacher. And ask them what AI is doing to assignments and tests. Disruptive Innovation (coined by Clayton Christensen) is the introduction of a product or service (often technology-based) into established markets, displacing traditional leaders via better and lower cost alternatives. (e.g. Uber & taxis, Facebook and Media etc.)
There is a lot of handwringing going on in my university about AI – specifically large, language models. Think Chat GPT, Bing, Bard and more.
I recently published a RCT using an AI-based clinical diagnosis app to determine how it can be integrated into high-stakes end of medical school national examinations. It was our attempt at answering a new version of the age-old debate on open-book exams. But I now worry that our research – published last month – is already out of date. Chat bots are disruptive innovation.
If you want a taste of what happens when SkyNET meets the National Board of Medical Examiners… well stay tuned!
Purpose
This study aimed to evaluate the performance of ChatGPT on questions within the scope of the United States Medical Licensing Examination Step 1 and Step 2 exams, as well as to analyze responses for user interpretability.
(Gilson et al., 2023)
Method
Two sets of medical knowledge questions were used to test the accuracy of Open AI’s chat bot – Chat GPT3.5 (As of this recording there is an updated paid version – Chat GPT4.)
- 100 questions from an exam bank (of thousands)
- 187 open access questions from the National Board of Medical Examiners.
- Questions addressed basic science (e.g. physiology, immunology etc.) and clinical practice (e.g. diagnostic criteria for a disease, management decisions) in medicine. (i.e. Step 1 and 2)
- There were 4 question banks (basic science X clinical practice X source)
- Only text-based questions were included (i.e. no image-based questions)
Data was entered manually, in a standardized fashion.
Chat GPTs reasoning and the use of internal (within the question stem) and external (beyond the data provided by the question) information was scored.
Chat GPT was compared against older-version chatbots.
Results/Findings
- Chat GPT correctly answered between 42 to 64% of the questions across the four question banks. In one data set, it achieved the passing score required of a third-year medical student.
- Chat GPT out performed earlier large language, natural langue processing models (e.g. GPT-3). These earlier models performed similar or worse than chance.
- As question difficulty increased ChatGPT’s accuracy dropped. However, this finding was not statistically significant in 3 of 4 datasets.
- ChatGPTS logical reasoning was consistent across (nearly) all questions in the two datasets, where this was studied.
- The necessary internal information from the stem was present in the answer (nearly) everytime with no statistical difference between correct or incorrect answers.
- Necessary external information (beyond the stem) was missing in incorrect answers between 27 and 42% of the time, compared to correct answers. This difference was statistically significant.
- Logical errors were most common, while tatistical errors (calculations, estimation of disease prevalence) were uncommon, in incorrect answers.
Conclusions:
“…our results suggest that ChatGPT performs at a level expected of a third-year medical student on the assessment of the primary competency of medical knowledge. Furthermore, the tool has potential as an innovation within the context of small group education … By providing sufficiently accurate dialogic responses akin to human learners, the model may facilitate the creation of an on-demand, interactive learning environment for students, which has the potential to support problem-solving and externally supported reflective practice.”
(Gilson et al., 2023)
The conclusion from the paper written by Chat GPT:
“Overall, this study suggests that ChatGPT has the potential to be used as a virtual medical tutor, but more research is needed to further assess its performance and usability in this context.”
What does this mean for us in HPE? The discussion is active now in all channels. Join the conversation here or on social media. We are looking forward to hear from you.
Chat GPT and assessment
Want to read up in some theory behind and tips? Arash Hadgadar and Andrew Maunder at KI has written up a summary of ChatGPT and assessment from their presentations on this spring’s “Bites of learning”
Ethical considerations on generative AI – join the discussion May 29
The Ethics Council at KI are welcoming to a seminar together with Unit for Teaching and learning. The hybrid seminar will be held in Swedish but will be recorded and subtitled for our English spoken associates.
More info and registration in the calendar invite.
Want more? Different aspects of AI in Medical education and Academia can be found in
Papers AI Theme Collection.
0 comments