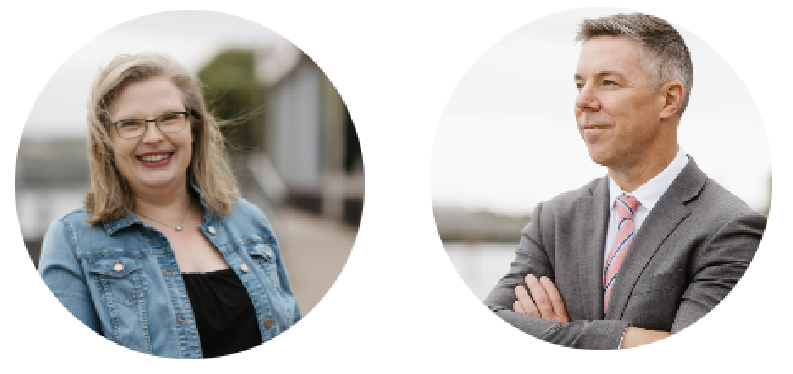
#54 – Methods Consult – Sample Size
Episode hosts: Lara Varpio and Jonathan Sherbino.
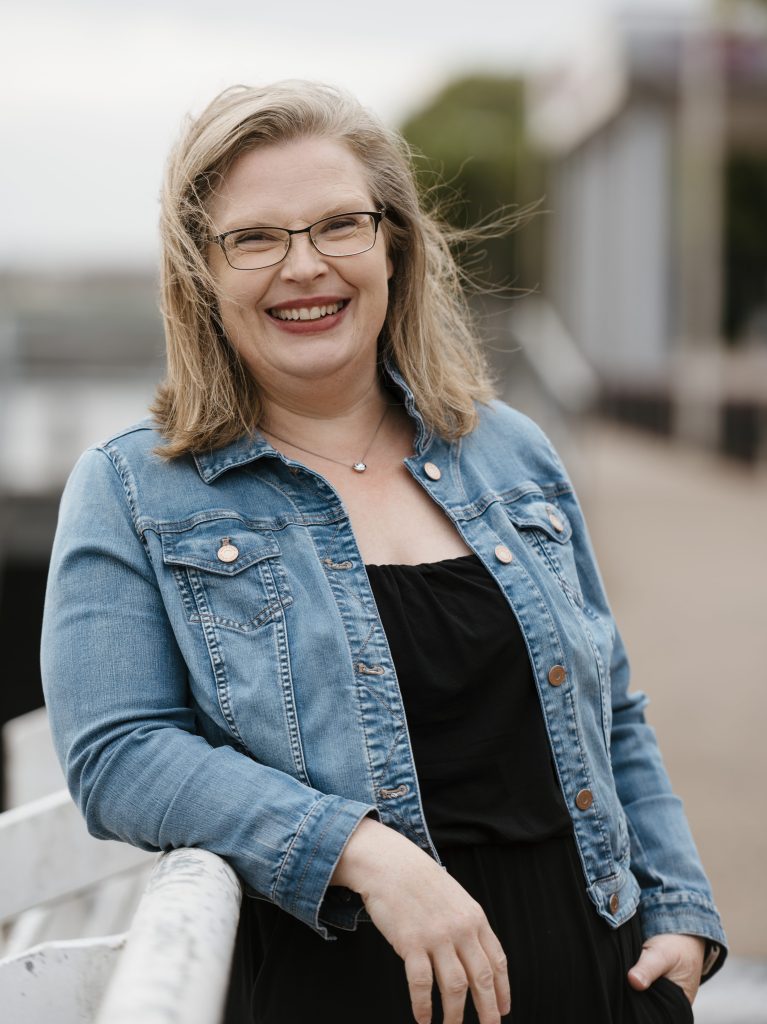
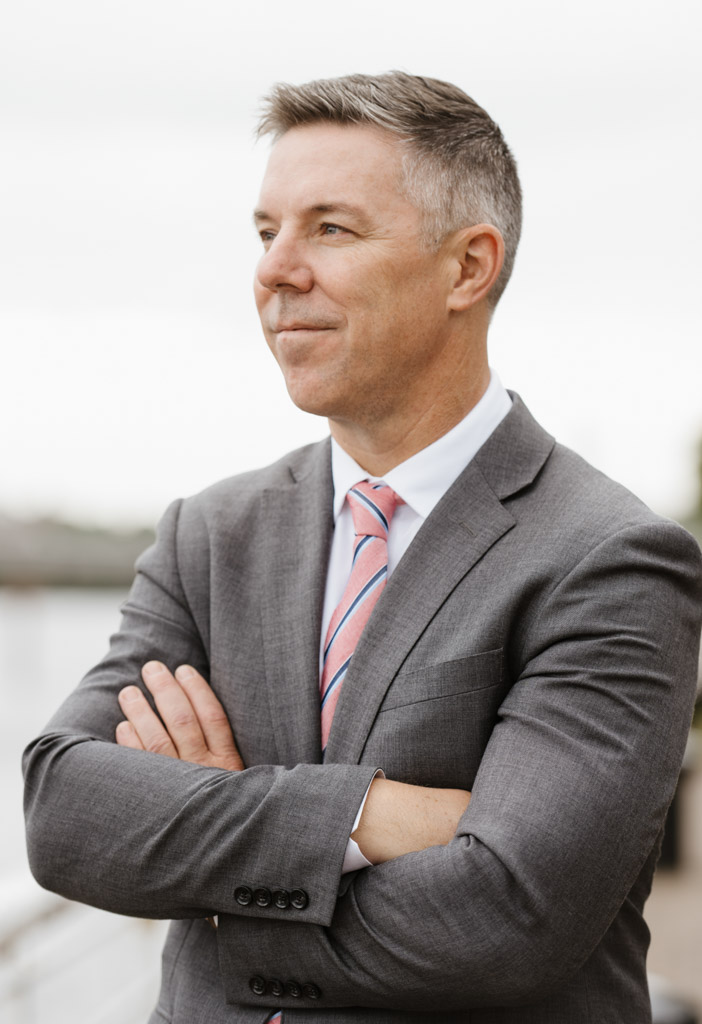
Sample size is a crucial aspect of research design, particularly in experimental studies. It influences the reliability and validity of the study outcomes. Here, Lara and Jon will break down some key concepts and methods related to determining and calculating sample size.
Episode notes
Why is Sample Size Important?
Sample size is crucial in research because it directly affects the validity, reliability, and generalizability of the study results. An appropriate sample size ensures that the study has sufficient power to detect meaningful effects or differences if they exist. Conversely, an insufficient sample size can lead to type II errors (failing to detect a true effect) and unreliable results. Besides that we can list two main reasons on calculating the right sample size:
- Ethical Considerations: In medical research, exposing participants to interventions carries ethical implications, especially if there could be potential harm. Hence, determining an appropriate sample size helps minimize unnecessary exposure.
- Resource Management: Conducting experiments is resource-intensive. An optimal sample size ensures efficient use of time, money, and effort without compromising the study’s integrity.
Quantifying Differences: Effect size measures the strength of the relationship between variables or the magnitude of an intervention’s impact. This is particularly important in education and health research where understanding the practical significance of an intervention is critical.
- Informing Sample Size: Calculating the required sample size for a study often involves specifying the expected effect size. This ensures the study is adequately powered to detect meaningful differences, thereby avoiding type I (false positive) and type II (false negative) errors.
- Comparability Across Studies: Effect sizes enable comparisons across different studies and contexts. This is especially useful in meta-analyses, where combining results from multiple studies provides a more comprehensive understanding of an intervention’s impact.
Power Analysis:
Power analysis helps in determining the sample size needed to detect an effect of a given size with a certain degree of confidence. The key elements include:
Power analysis is a statistical method used to determine the minimum sample size required to detect an effect of a given size with a desired level of confidence. The key parameters for power analysis are:
Key Concepts
- Type I Error (α Error): Incorrectly rejecting a true null hypothesis (false positive).
- Type II Error (β Error): Failing to reject a false null hypothesis (false negative).
- Power: The probability of correctly rejecting the null hypothesis when there is a true effect. Typically, researchers aim for a power of 0.8 (80%), meaning there’s an 80% chance of detecting an effect if there is one. Higher power reduces the risk of a Type 2 error, which is missing a real difference.
- Alpha (α): The significance level, often set at 0.05, which is the probability of a type I error (false positive). It indicates a 5% risk of concluding that there is an effect when there actually isn’t one.
- Effect Size: This represents the magnitude of the difference between groups in a study.termining sample size; larger effect sizes require smaller sample sizes to detect, while smaller effect sizes require larger sample sizes. Common effect sizes include Cohen’s d, which expresses the difference between two means in terms of standard deviations.
Different research fields might prioritize different aspects when calculating sample size. In biomedical research, stringent ethical considerations often dictate larger sample sizes to detect even small effects due to potential risks to participants. In education research, practical constraints such as limited resources often lead to smaller sample sizes.
Practical Application
- Example Study: If you are comparing a new teaching method using a simulator against traditional methods, you need to decide on the expected effect size based on previous literature or pilot studies.
- Randomization: Ensuring participants are randomly assigned to control or intervention groups helps mitigate biases and makes the groups comparable.
- Convention and Feasibility: Due to resource constraints, many educational studies opt for large effect sizes, which require smaller sample sizes. However, this might not always be ideal or realistic.
Pilot Studies: Conducting a pilot study to gather preliminary data helps estimate the effect size more accurately and adjust the sample size calculations accordingly.
References
- Cook, D. A., & Hatala, R. (2015). Got power? A systematic review of sample size adequacy in health professions education research. Advances in health sciences education: theory and practice, 20(1), 73–83.
This paper discusses the importance of power and sample size calculations, with a focus on the health professions education context.
- Norman, G. (2010). Likert scales, levels of measurement and the “laws” of statistics. Advances in health sciences education: theory and practice, 15(5), 625–632.
This article provides insight into the appropriate use of statistical measures in health professions education research, including effect size.
0 comments